I don’t miss the exploding-head emojis everywhere but it sure feels like the hype around AI has toned down a bit. It may just be that I curate my news feeds better. Or that Donald Trump takes up too much space in the media. But it can certainly be partially explained by concentration dynamics in every segment of the AI market.
This concentration is not so much a consequence of acquisitions - although acqui-hires continue to be a theme - but rather evidence that wannabe entrepreneurs have moved on after failing to find product market fit or, if they did find it, after seeing their product commoditized. It’s hardly a surprise; this very blog addressed the winner-take-all dynamics on several occasions last year.
Google is now exactly where it was expected to be: at the top of the model rankings. But its technical leadership has not yet been converted into a commercial leadership as OpenAI is still very much ahead on that front. And seemingly accelerating.
Before addressing OpenAI’s peculiar strategy to get to 1 billion users (hopefully not before I manage to post this blog), let’s focus on corners of the AI world that are still very dynamic. In some segments, it still feels like 2023. One of these segments is what I call "small reasoning engines", i.e. small LLMs (fewer than 3 billion parameters) trained to have strong reasoning and/or tool-use capabilities. But writing about these models seems premature : there are signs that it may work (I want to believe) but their usefulness is still limited for real life use cases.
TTS and STS
At the end of 2023, this blog reviewed the best solutions for various modalities, commenting on the huge progress expected across the board for 2024. It did not really happen. The most impressive evolution was probably in video, but it mostly concerned closed-source models (Wan2.1 was only published in 2025).
The lack of significant progress in the voice modality (text-to-speech or speech-to-speech) was a surprise. All the more so because Coqui, the best open-source solution in 2023, was shut down.
In that context, it was equally surprising to see a Cambrian explosion of speech models in early 2025. This explosion wasn’t kick-started by Big Tech companies but by small labs. The models are open-source and they are small so they run at the edge. The toothpaste is out of the tube ; this ecosystem is evolving very quickly. And this has consequences for other segments.
Notable releases include :
- Hibiki: speech-to-speech translation with voice cloning, 1-billion and 2-billion parameter models.
- Kokoro: text-to-speech, 82m parameter.
- CSM: speech-to-speech and text-to-speech. 1-billion parameters
- Dia: text-to-speech, handling dialogs. 1.6-billion parameters
A true market standard has yet to emerge and it is currently fairly difficult to switch between models. For Mac users, I'd recommend mlx-audio for simple experiments with the various models. Notwithstanding the lack of market standards, the speech modality can be considered solved. And these small models exceed what Amazon (Alexa) or Apple (Siri) offer.
Apple
Is it a problem for Apple? The optics are not great but it could easily be turned into an opportunity: if third-party apps, sold on the App Store, monetize this service, Apple would get a cut of the proceeds. That said, the recent ruling by the Court of California (Apple vs. Epic) prompted Apple to update its terms and allow external links - and therefore allow payments outside the Apple platform - for apps downloaded from the US app store. It now seems likely that other jurisdictions will push Apple to do the same for their regions which, combined with the new tariffs imposed by the US government, constitutes a perfect storm for the company’s finances.
Coming back to third-party voice models offered on iPhones : for users, it’s hard to believe that the service could be as good as an integrated solution by Apple. There are also privacy and mental health considerations for users, in particular, if the services are free.
For Boomers and Gen-Xers, the latency of current voice models may still be problematic as the dialogue can feel uncanny. But younger generations seem accustomed to asynchronous communication via voice memos and AI voice-over in social media content is increasingly prevalent. So product market fit may not be so distant. Voice quality definitely isn’t an issue, you can now convincingly simulate Scarlett Johansson’s voice like in Her (2013).
Sycophancy
The widespread adoption of speech-to-speech models would raise concerns regarding users' mental health. Without going as far as falling in love with an AI like Joaquin Phoenix in Her, interactions in this modality are not neutral as they can activate our brain’s natural reward/reinforcement mechanisms just like those that get you hooked on your social media feeds (and more recently the short-form video format). To be clear, however, the small STS models that currently run on the edge are unlikely to ensnare users : voice alone is not enough, you’d need a strong model to fully conquer users’ brains (more parameters, more training data and a bit of post-training magic).
Here comes GPT-4o, OpenAI’s mainstream model. It had been obvious for months that the model was fine-tuned to maximize user engagement. Whether or not this was voluntary is still unclear but it was, at the very least, a consequence of including user feedback (thumbs up or down) in the post-training phase. This strategy also tend to reward agreement with users, amongst other side effects like cancelling the Croatian language (see screenshot below). The sycophantic collapse reached a tipping point in April, triggering a public backlash and prompting OpenAI to roll back the latest model update.
To OpenAI's credit, they did acknowledge the mistake - albeit user engagement remains an objective1 - and they published a fairly detailed post on the origins of the issue. Would they have disclosed so much information about the release process if the new model had only been slightly more sycophantic than the previous iteration? It was noticeable this time because it was such a significant step-change, but the trajectory had been locked-in for a while (and there is literature on this type of model behavior).
Read the account of this controversy in a recent Interconnect newsletter.
OpenAI
OpenAI’s transparency and swift reaction almost came as a surprise given they have often seemed to prioritize [prospective] shareholders and regulators over customers’ interests. The handling of this controversy dubbed the 'glazegate' is the latest evidence of a shift in focus that has happened in the last few months. The focus is now on customers with a unique product strategy that is, somewhat unexpectedly, working.
An important backdrop to the apparent shift is that 1/ OpenAI secured a $50bn funding round led by Softbank so they probably don’t need to worry about prospective shareholders for a while and 2/ OpenAI has received public support of the US government which got directly involved in the fundraising effort to build infrastructure to support their operations2.
The maximization of user engagement and retention is now fully baked into OpenAI's product strategy : the best models are only available in a cost-efficient manner through their platform. When they are available via API, they are stupidly expensive. OpenAI also creates scarcity through rate-limits but it’s hard to say whether it is a way to manage GPU capacity or purely a marketing strategy. In any case, they don’t seem to have demand issues and they try to funnel this demand through their web app3.
The true value-add of their own platform does not only stem from the fact that the best models are available there as part of the subscription plan (with different rate limits depending on subscription level) but also because they are integrated in systems (agents) that work incredibly well. Or at least better than what you could reasonably expect to achieve if you called the models directly and tried to build an agentic system around the responses. It works well for OpenAI because the post-training is designed with the product in mind - that’s also the origin of the sycophantic collapse. The model is the product. And OpenAI shows that they actually have product expertise. My criticism last year is aging terribly : while I can’t say that I agree with the product philosophy (social-media-like) I have to acknowledge that, in terms of execution, the product is vastly superior to what competitors offer.
The Rest of the Frontier
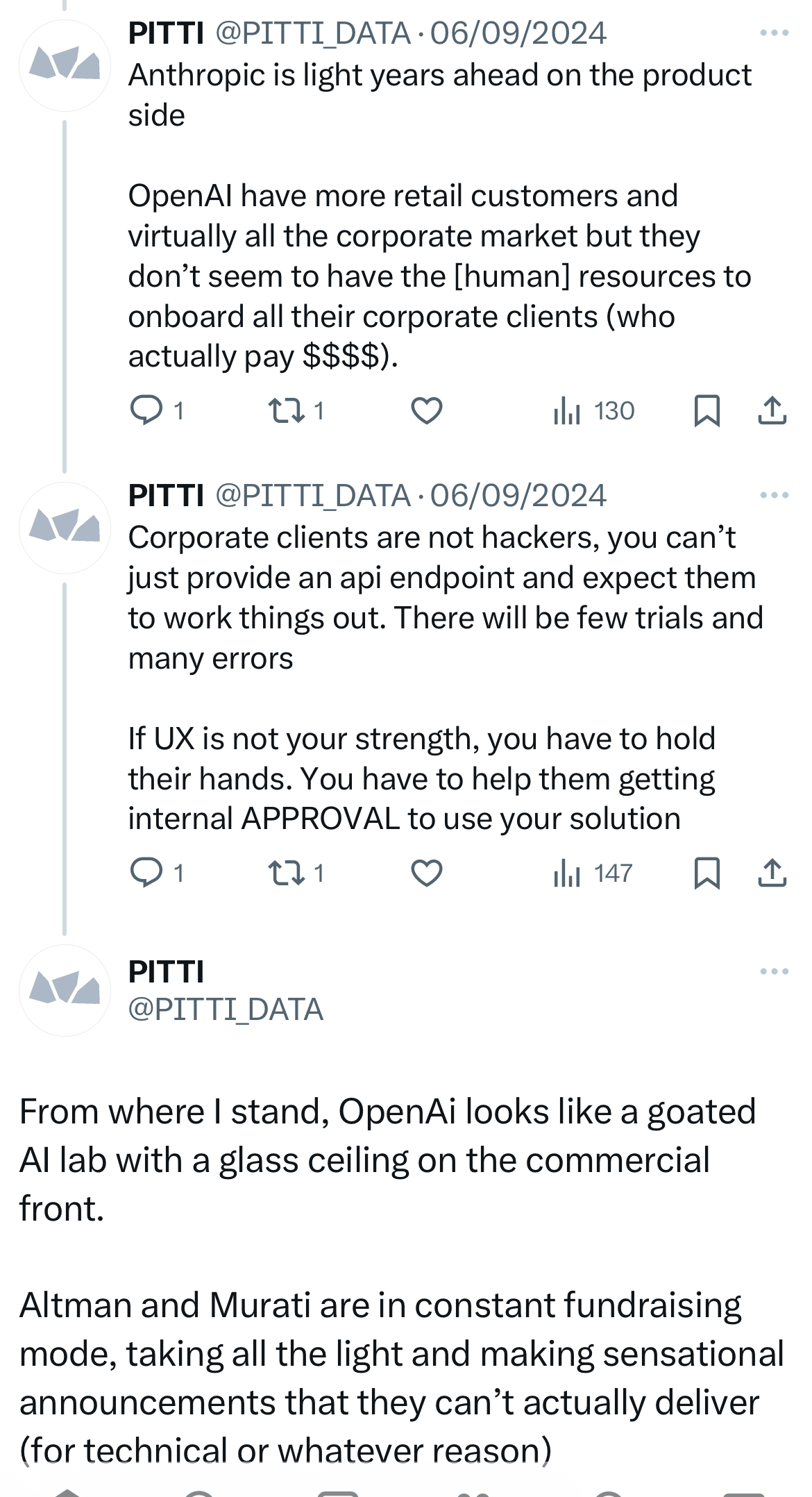
Last year’s criticism of OpenAI’s product expertise is also aging terribly because it put this expertise in perspective with Anthropic’s, which seemed, at the time, far greater. In hindsight, there are many reasons why this was an incorrect assessment of Anthropic’s true capabilities. There were plenty of generally good ideas4 - I still personally believe that the split window with artifacts presented of the right is the way to go for AI assistant web apps. The problem is that it only worked as a product with Claude Sonnet 3.5 and 3.5(new). In 2025, the product is extremely hard to use because 1/ Anthropic seems helpless regarding their network reliability issues and 2/ Claude Sonnet 3.7’s willingness to go above and beyond what was asked - sometimes ignoring instructions in the process- is often annoying. I now exclusively use Sonnet for tasks including visual representations or visual understanding. This means that I use around 1 million tokens a month and the subscription is just not worth it; the API will do.
If you want to integrate LLMs in your software stack, APIs remain the best option. Firstly because running frontier models like Deepseek's requires multiple Nvidia GPUs which is not only expensive but also very complex. Frontier labs also provide peace-of-mind solutions with their standardized APIs (increasingly converging towards the OpenAI standards) set up by experts and relatively well documented. But it is fair to say that these APIs still aren’t on par with what you’d get in other fields. This thread on X gives a good overview of the difficulties that developers face. So on the one hand, we see AI startups finally focusing on the product and on customers instead of funding rounds, which is a mark of maturity for the sector. But on the other hand, we are still very far from an acceptable level of service for customer retention in the most profitable segment of all : B2B using APIs to automate processes.
Google’s strategy is interesting in the sense that they always seem reactive on the product side. They do not innovate; they replicate what works. As if they learnt from the errors of the past (Gemini 1 benchmarks, woke image generation…) and do not take any risks. They now have the best models out there, at very competitive prices, and they gradually remove frictions to using the models so that market forces can play out in full swing.
Model Personality
An area where Google really doesn’t take any risk is model personality. This is a divisive subject. I personally like the Google approach, where the model is basically a tool and its personality is that of an "autistic engineer"5 but I seem to be part of a minority. The community seems to agree that a non-neutral personality is critical for commercial success. It was arguably a dimension of Claude Sonnet’s rise in 2024 as many users started to use the model for mere chatting and praised its personality.
OpenAI’s strategies to increase user engagement directly speak to model personality and do not solely revolve around tricks like asking follow-up questions. As it is clear that there is no one-size-fits-all personality, OpenAI models take into account the user's chat history to steer the model’s tone at inference time. Assuming that personality really is a differentiator, OpenAI’s "memory" feature is another way to make their platform more compelling than the API. As part of the 'glazegate', we learnt that the summary of each user's profile based on memories was passed to the model but that OpenAI had to hide the summaries to avoid hurting users’ feelings. This is, in itself, an interesting illustration of the tension that can exist between user experience and quality of service.
1/ OpenAI employee commenting on the side effects of reinforcement learning
2/ Changes in GPT-4o's system prompt after the 'glazegate'
3/ OpenAI employee commenting user profiling
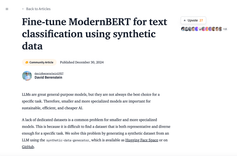
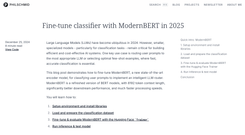
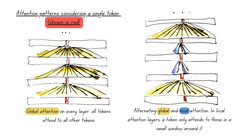
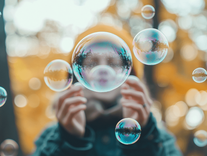
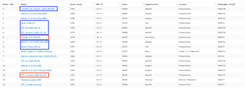