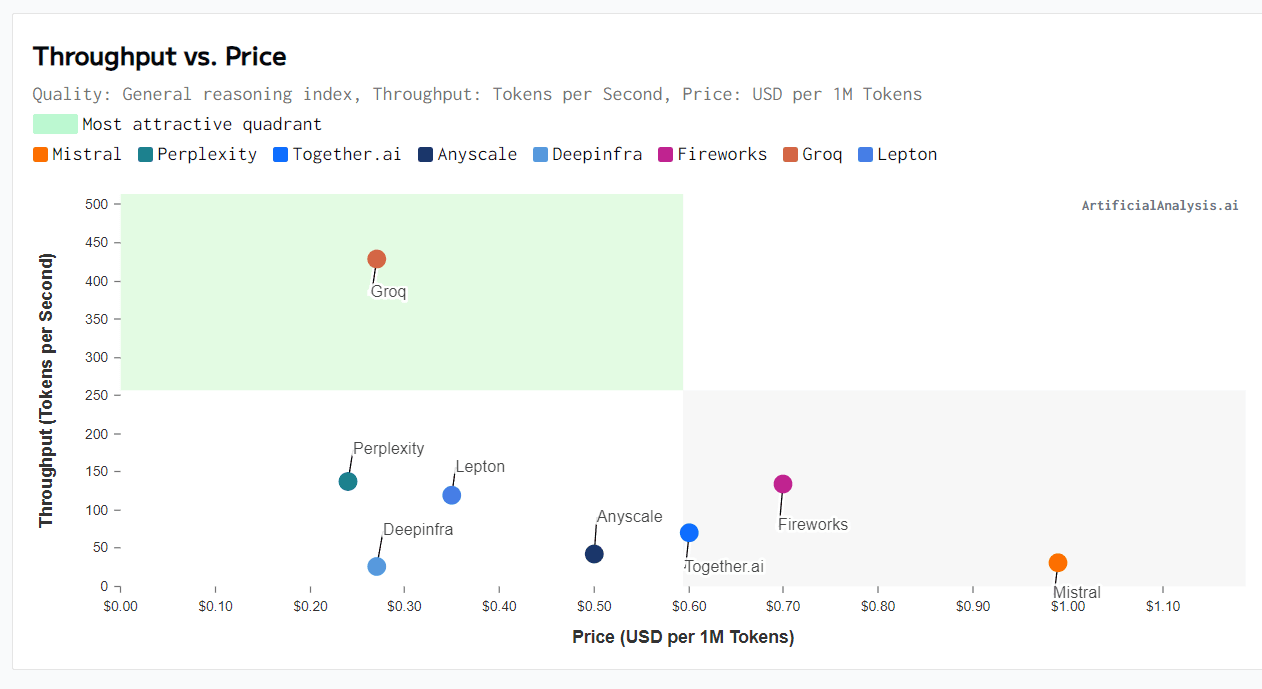
Description
Groq, an AI hardware startup, has been making the rounds recently because of their extremely impressive demos showcasing the leading open-source model, Mistral Mixtral 8x7b on their inference API. They are achieving up to 4x the throughput of other inference services while also charging less than 1/3 that of Mistral themselves. However, speed is only one part of the equation. Supply chain diversification and total cost of ownership (TCO) are also crucial factors to consider. Groq's chips are entirely fabricated and packaged in the United States, giving them an advantage over competitors who rely on memory from South Korea and chips/advanced packaging from Taiwan. However, the primary formula for evaluating if hardware is revolutionary is performance / total cost of ownership (TCO). Google understands this intimately, as their infrastructure supremacy is why Gemini 1.5 is significantly cheaper to serve for Google vs OpenAI GPT-4 Turbo while performing better in many tasks, especially long sequence code. Google uses far more chips for an individual inference system, but they do it with better performance / TCO. Performance in this context isn't just the raw tokens per second for a single user, i.e., latency optimized. When evaluating TCO, one must account for the number of users being served concurrently on hardware. Most edge systems won't make up for the increased hardware costs required to properly run LLMs due to such edge systems not being able to be amortized across massive numbers of users. As for serving many users with extremely high batch sizes, IE throughput and cost optimized, GPUs are king.
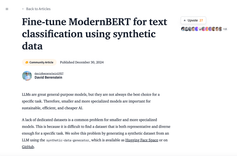
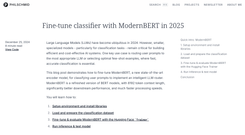
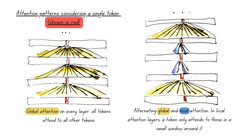
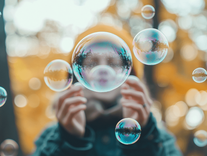
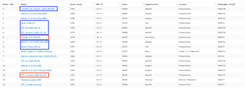